PredXGBR
PredXGBR A Machine Learning based Short term Electrical Load Forecasting Architecture
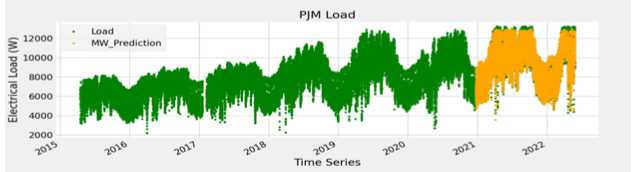
The increase of consumer end load demand is leading to a path to the smart handling of power sector utility. In recent era, the civilization has reached to such a pinnacle of technology that there is no scope of energy wastage. To prevent both electricity shortage and wastage, electrical load forecasting becomes the most convenient way out. Artificial Intelligence, Conventional and Probabilistic methods are employed in load forecasting. However, the conventional and probabilistic methods are less adaptive to the acute, micro, and unusual change of the demand trend. With the recent development of Artificial intelligence, machine learning has become the most popular choice due to its higher accuracy based on time, demand, and trend-based feature extractions. The proposed model considers the extensive features derived from short-time lag-based autocorrelation. Also, for an accurate prediction from these extracted features, few machine-learning models have been employed from previous research works. Among the effective most models, Recurrent Neural Network (RNN-1 and RNN-2) and Long-Short Term Memory (LSTM-1 and LSTM-2) models are employed for forecasting daily load demand. Followed by two Extreme Gradient Boosting (XGBoost) Regression based models: (i) PredXGBR-1 and (ii) PredXGBR-2 have been proposed with both definite long, and short-time lag feature to predict hourly load demand. Apparently, the short time lag feature employed to XGBoost regression algorithm provides the best outcome with an MAPE of around 1.5%-2%. The proposed model is validated with five different historical data record of eastern and western zone of USA over twenty years of time span. Followed by the validated model with satisfactory accuracy is employed to Power Grid Company Bangladesh (PGCB) historical dataset to build load forecasting architecture of Bangladesh. The R2 value of PredXGBR-1 and PredXGBR-2 are 0.89475 and 0.9579 with an average MAPE (error) of 15.11% and 2.73% respectively. Apparently, we propose the definite short time lag featured PredXGBR-2 over the other models for the forecasting architecture considering the performance evaluation.